Modeling, Simulation and Optimization of Process Chains
- Institute:
Lehrstuhl Angewandte Mathematik 2, FAU Erlangen-Nürnberg
- Project Leader:
Prof. Dr. G. Leugering, FAU Erlangen-Nürnberg
- Research Assistant:
- M.Sc. L. Pflug, FAU Erlangen-Nürnberg
M.Sc. M. Spinola, FAU Erlangen-Nürnberg
Project Goal
The mathematical description of whole process chains by flow sheets as well as their numerical implementation, for instance within DYSSOL, allow their holistic simulation. Based on this, model-based optimization with regard to desired properties of products and energetic aspects can be applied. The development of disperse properties during the process as well as the relation between disperse properties and the properties of the final product (e.g. absorption spectra, flow properties) have to be modeled mathematically in order to allow a model-based optimization of the product properties w.r.t. the process parameters (e.g. residence times, process temperatures, flow velocity, feedback rate, selectivity of screens, milling capacity).
This subproject aims to derive a mathematical model of process chains as well as to perform a sensitivity analysis of the real problems which are considered in this DFG priority program. The processes which are modeled in the mentioned problems mostly base on population balance laws (PBEs) which are coupled with algebraic equations.
Results
From the mathematical point of view, PBEs are nonlinear, nonlocal transport equations which allow a balancing of the number concentration w.r.t. intern variables (e.g. length, diameter) over time. For real process chains the whole system has to be represented mathematically. The general form involves the following nonlinear partial differential equation with given initial and boundary data
$$\begin{align*}
\dot{q}(x,t)+\partial_x\big(R[W[q,\gamma,a,b]](x,t)q(x,t)\big) &= h[q](x,t) && t>0,x\in[x_{\min},\infty)\\
q(x_{\min},t) & = n(t)\\
q(x,0) & = q_0(x)\\
W[q,\gamma,a,b]&= \int\limits_{a(x)}^{b(x)}\gamma(t,x,y)q(y,t)\text{d} y.
\end{align*}$$
In the context of a mathematical analysis of the upper equations, the already existing theory regarding nonlocal transport equations was problem-specifically extended such that also nonlocalities in the source terms (e.g. feedback by mills and screens within a spray granulation process chain) as well as ripening velocities depending nonlocally on the disperse properties (e.g. total surface, total mass in the particle size distribution) can be considered. Via the method of characteristics and fixed-point arguments it was possible in a joint work with Dr. A. Keimer (EECS, Berkeley) to set up conditions which guarantee the existence of a unique and sufficiently regular solution.
Based on the mentioned analysis, a numerical method was realized which could be applied, among others, on the study of spray granulation processes. For this numerical implementation the nonlocal partial differential equation was transformed to a nonlinear system of ordinary differential equations by use of the method of characteristics and by discretizing the disperse properties. This transformation facilitates the construction of an efficient numerical method by using a time-discretization scheme as well as an adaptive discretization in the spatial and time variable.
In view of the calculation of sensitivities, it was possible not only to simulate the given process but also to simulate and to verify the adjoint PBE after prescribing an objective functional. Thereby, within this subproject C5 the derivation of the adjoint equation occured by applying the so-called Lagrange formalism w.r.t. time-dependent control parameters. The implemented first order optimization method based on the previous procedure was exemplarily, in cooperation with the working group Heinrich (A3,Z), applied on a PBE which models a spray granulation process coupled with a filtered and milled feedback. Thereby, the time-dependent parameter to control corresponds to the inflow rate of new nuclei. As you can see in fig. 1, you can goal-orientedly influence the dynamic process by controlling the mass flow. Moreover, as illustrated in fig. 2, the mass to be milled can be reduced by choosing an optimal constant control. Furthermore, an iterative calculation of a time-dependent locally optimal control shows that the previous mentioned mass can be diminished even more. This mass reduction evidently decreases the energy you have to spend for it.
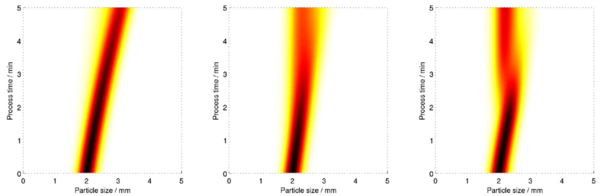
Within the scope of a simulation and optimization of micro reaction plants considerd within the DFG priority program, the simulation tool FIMOR (Fully Implict Method for Ostwald Ripening), which was developed in our research group, was exploited in order to perform in subproject A8 (working group Peukert) model-based parameter studies w.r.t. the different control parameters. Due to the efficient implicit implementation and the low number of control variables, it was possible to establish within a cooperation of working group Peukert and working group Leugering optimal process conditions for the desired product attributes respectively without using gradient information. Moreover, some model-based optimization results could be realized on a micro reaction plant considered within subproject A8.
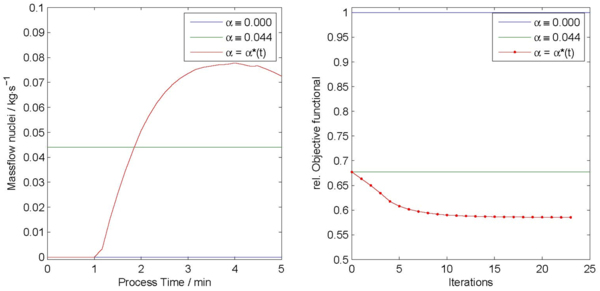
Further work program
The fundamental mathematical model which yields the basis of the sensitivity analysis will be extended such that PBEs with temporal nonlocalities in the source terms can be analyzed in order to depict a feedback within a process chain. This extension is motivated by the presence of a feedback within a process chain which requires a certain time period to be accomplished or which underlies a residence time distribution. Also in the modeled form, this residence time can depend on the disperse property and thus its detailed analysis will represent a generalization of the results obtained in [Spinola 2017].
With regard to possible controls (source terms, ripening functions, initial and boundary conditions), the to the considered PBE corresponding adjoint equation will be derived as general as possible. Its solution allows together with the simulation of the process to calculate gradients of objective functionals. This procedure will exemplarily be verified for problems which are relevant for the DFG priority program.
Finally, an interface file (HDF5-file) developed within the Z-project (working group Heinrich) in Dyssol provides the possibility to calculate the gradient of the objective functional w.r.t. time-varying control parameters by simulating two flow sheets.